Spector Receives NSF Grant To Propel Computer Programs Modeling Biological Evolution
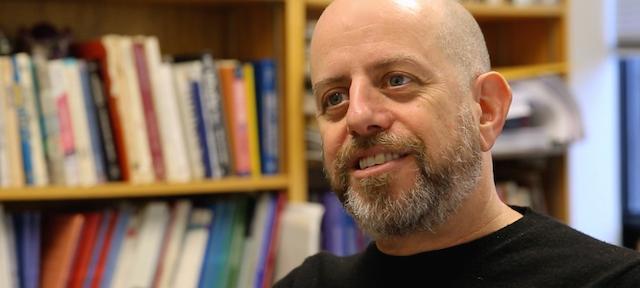
It took a few billion years of evolution for single-cell organisms to evolve into multicellular plants and animals. But only seven decades after humans proposed early designs of artificial intelligence and machine learning, Hampshire Professor of Computer Science Lee Spector is trying to propel computer programming forward by developing programs that not only evolve, but also evolve as innovatively as biological evolution itself. Spector has now received a three-year grant of $419,000 from the National Science Foundation — his second major NSF grant exceeding $400,000 in six years — for research and development of this technology. These grant programs in NSF’s Information & Intelligent Systems Division are highly competitive, funding just 15 percent of proposals in 2015.
This cutting-edge work attempts to replicate the process of evolutionary biology in computers to solve problems more quickly and more innovatively than humans can. Over billions of years, evolution has resulted in radical, brilliant adaptations of living things, including humans, Spector says. He is co-leading a research group called the Hampshire College Computational Intelligence Lab, in which students and scientists from several institutions, from the Five Colleges and beyond, regularly participate. “We’re trying to model nature’s tricks and secrets that have produced all the marvelous features of the natural world and life,” he said, “and use them in computers to be creative and innovative like nature.”
Over the past decade, computational evolution has begun to show real progress. Spector and other scientists have created programs that are able to use the basic evolutionary model of random variation and selection to produce designs, algorithms, and other solutions that are equal to or more advanced than what humans are capable of.
These computer-based problem-solving systems are already revolutionizing areas of science and engineering, with impacts on economic activity, human health, national security, and science. The field’s annual awards, the HUMIES, recognize work that has generated a demonstrably human-competitive solution, among them ones resulting in patents or that may qualify for a patent.
A couple years ago, Spector and six other scientists analyzed 42 computer programs that had received a HUMIE. They reported that these computer programs have been used to solve difficult problems in, for example, physics, computer science, mechanical and software engineering, mathematics, medicine, and games.
The most publicized product has been an “evolved antenna” used for a 2006 NASA mission, Space Technology 5. An evolutionary computation system used random variation and selection to produce the best design for the antenna. The final design, resulting from the highly complex data requirements, was arguably beyond the ability of humans to accomplish. A Wikipedia entry calls the antenna “the world’s first artificially evolved object to fly in space.”
In another application, Spector and a team of five scientists have been leading a study to model how wind turbines behave, in order to improve their operation. “The models produced by human engineers are usually pretty bad,” Spector says, “so we’re using computers to better evolve the sets of equations that model the behavior of the turbines.”
But what is so far beyond the reach of Spector and his peers is the ability to develop these programs to the next stage: in which they will evolve toward improving their own random variation and selection algorithms, while they work, without human involvement.
Evolutionary computing scientists use ancestry trees to visualize their program's progress seeking solutions to problems.
The NSF grant will enable Spector to focus squarely on one of the most exciting and radical ideas that have come out of their research group in the past 15 years. Despite work by thousands of computer scientists, Spector says none of them has yet produced the kind of radical innovations that are characteristic of biological evolution. But he’s now hopeful, and he’s helping to lead the charge.
“Biological evolution using random variation and natural selection is a designer,” he said. “The way humans produce children is not like the way bacteria or mosquitoes produce children. What we’re trying to do is to get evolution to happen in computers so that the way in which programs produce other programs itself evolves.”
The evolution in evolutionary computing can be described as a two-step repetitive process of random variation and then selection: A computer program searches for a solution to a problem, or designs a solution to a problem, by varying and mutating and testing hundreds, thousands, or millions of variations of solutions. Those solutions found most fit and effective are selected by the program to “survive” and to be further varied and tested until a final workable design or solution is found.
Spector elaborates: A program may produce, after many generations, genuinely novel and useful designs and inventions. Although differences remain between the processes of biological evolution and evolutionary computing, they’re both fundamentally driven by random variation and selection, he said, “and the successes of one hint at the power of the other.”
For his three-year, NSF-funded project, Spector has proposed that evolutionary-computer technology is on the brink of being able to evolve with the potential to solve even more-difficult problems. He will begin his research project with a promising system of this type and will test it systematically toward developing more-refined, adaptive algorithms. He will also integrate research and education across undergraduate and graduate levels, providing training to a new generation of computer scientists.
This NSF grant helps to fund Spector’s group, specifically his own summer research, graduate-student research at UMass, and travel to conferences. Spector frequently invites Hampshire and Five Colleges undergraduates and UMass graduate students to collaborate on this research, coauthor papers, and present together at global conferences. Hampshire undergrads can actively get involved as early as their first year of college and can quickly become full-fledged collaborators, rare in undergraduate education.
A number of Spector’s students from Hampshire and UMass presented with him this summer at the global Genetic and Evolutionary Computation Conference, this year held in Denver. One presenter was first-year Hampshire student Julian Oks, who got involved in the research group in his first semester and months later was coauthor with Spector and researchers from the University of Minnesota and Washington and Lee University on a paper titled “Evolution Evolves with Autoconstruction.”
In it the researchers report that they have developed a programming model (which Spector named “autoconstruction”) that has proved in testing to solve some difficult problems and to begin to evolve in the way individual solutions to a problem automatically create variations of offspring solutions.
Another Hampshire student who was involved in the research group is recent graduate Eddie Pantridge, who also attended the July conference to present his Division III thesis research, based on computational evolution. Spector was chair of his committee. Pantridge is now in the Mass Mutual Data Science Scholars program working as a data scientist while pursuing a master’s at UMass.
For these faculty–student collaborations, Spector has been recognized with a National Science Foundation Director’s Award for Distinguished Teaching Scholars. He has coauthored with undergraduates more than a dozen articles and reports (see list below of publications co-authored by Hampshire students).
Another version of an ancestry tree.
Spector — editor in chief of the journal Genetic Programming and Evolvable Machines — is widely recognized as a leading researcher in the field. The Association for Computing Machinery has recently established the Special Interest Group on Genetic and Evolutionary Computation (ACM SIGEVO), and invited Spector as the only professor from an undergraduate college to serve on the executive board. In addition to teaching in Hampshire’s School of Cognitive Science, Spector is on the faculty of the College of Information and Computer Sciences at UMass Amherst.
Spector and his research group of other scientists and students in the Hampshire College Computational Intelligence Lab meet once a week via video chat to discuss one another’s work and their collaborations. The group is a global leader in the field. (See their discussion site: https://push-language.hampshire.edu). Members operate a high-performance computer cluster called fly, which has 768 processing cores in it, a significant level of computing power.
Spector says one area of innovation for his research group is to write computer programs that automate what human computer programmers do. Instead of hiring a human programmer to write a computer program, you give a computer a description of what you want the program to accomplish and the computer, through its evolutionary-computing process, finds the best selection for the challenge.
“We’re far from that,” he says, but he envisions a day perhaps in the next decade when consumers can use an app store to specify what they want and a computer will automatically program a new app.
One way he and his peers analyze progress in their work is by using virtual beings or agents in virtual worlds. Computer scientists program virtual beings to evolve in simulated worlds that resemble video-game design. With his proposed NSF project, Spector will use a new virtual-world environment he developed called “Pucks” (video http://blog4pucks.blogspot.com). He has used Pucks both for research and education and in two of his undergraduate courses on artificial intelligence, to teach and study complex behaviors and interactions.
A screen grab from Lee Spector's virtual-world environment, Pucks.
Spector’s student collaborator Eddie Pantridge was one of the contributors to the development of Pucks. “Evolutionary computing has the potential to improve the lives of a lot of people,” said Pantridge, who graduated in May. He hopes to one day use the new technology to develop new applications that will enable medical professionals to diagnose and treat illnesses in remote locations, such as in rural communities and in developing countries. “It sounds like sci-fi,” he said, “but it’s within our grasp.”
Led by pioneers such as Spector and the many students he’s inspired, this computational-evolution technology holds the promise not only to remake research and development of products across industries, but also to remake life, and artificial life, as we know it.
Lee Spector, Publications with Undergraduates, through Sept 20, 2016
* = undergrad co-author ** = Hampshire undergrad co-author
Journal/Conference/Workshop Papers
Helmuth, Thomas, Lee Spector, Nicholas Freitag McPhee, and Saul Shanabrook*. Linear Genomes for Structured Programs. In Worzel, William, William Tozier, Brian W. Goldman, and Rick Riolo, Eds., Genetic Programming Theory and Practice XIV. New York: Springer.
McPhee, Nicholas Freitag, Mitchell D. Finzel*, Maggie M. Casale*, Thomas Helmuth and Lee Spector. A detailed analysis of a PushGP run. In Worzel, William, William Tozier, Brian W. Goldman, and Rick Riolo, Eds., Genetic Programming Theory and Practice XIV. New York: Springer.
Kannappan, K., L. Spector, M. Sipper, T. Helmuth, W. La Cava, J. Wisdom**, and O. Bernstein**. 2015. Analyzing a decade of Human-competitive ("HUMIE") winners -- what can we learn? In Genetic Programming Theory and Practice XII, edited by R. Riolo, B. Worzel, and M. Kotanchek, pp. 149-156. New York: Springer.
Spector, L., K. Harrington, B. Martin**, and T. Helmuth. 2011. What's in an Evolved Name? The Evolution of Modularity via Tag-Based Reference. In Genetic Programming Theory and Practice IX, edited by R. L. Riolo, E. Vladislavleva, and J. Moore, pp. 1-16. New York: Springer.
Helmuth, T., L. Spector, and J. Matheson**. 2014. Solving Uncompromising Problems with Lexicase Selection. In IEEE Transactions on Evolutionary Computation. DOI: 10.1109/TEVC.2014.2362729.
Spector, L., J. Klein, and K. Harrington**. 2005. Selection Songs: Evolutionary Music Computation. In YLEM Journal, Vol. 25, No. 6 & 8, pp. 24-26 (associated web page, including link to full text).
Spector, L., and A. Robinson**. 2002. Genetic Programming and Autoconstructive Evolution with the Push Programming Language. In Genetic Programming and Evolvable Machines, Vol. 3, No. 1, pp. 7-40.
Pantridge**, E., and L. Spector. 2016. Evolution of Layer Based Neural Networks: Preliminary Report. In Companion Publication of the 2016 Genetic and Evolutionary Computation Conference, GECCO'16 Companion. ACM Press. pp. 1015-1022.
Spector, L., N. F. McPhee, T. Helmuth, M. M. Casale*, and J. Oks**. 2016. Evolution Evolves with Autoconstruction. In Companion Publication of the 2016 Genetic and Evolutionary Computation Conference, GECCO'16 Companion. ACM Press. pp. 1349-1356.
McPhee, N. F., M. M. Casale*, M. Finzel*, T. Helmuth, and L. Spector. 2016. Visualizing Genetic Programming Ancestries. In Companion Publication of the 2016 Genetic and Evolutionary Computation Conference, GECCO'16 Companion. ACM Press. pp. 1419-1426.
Spector, L., B. Martin**, K. Harrington, and T. Helmuth. 2011. Tag-Based Modules in Genetic Programming. In Proceedings of the Genetic and Evolutionary Computation Conference (GECCO-2011). ACM Press. pp. 1419-1426.
Helmuth, T., L. Spector, and B. Martin**. 2011. Size-Based Tournaments for Node Selection. In GECCO'11 Workshops, Genetic and Evolutionary Computation Conference. ACM Press. pp. 799-802.
Spector, L., D. M. Clark, I. Lindsay**, B. Barr**, and J. Klein. 2008. Genetic Programming for Finite Algebras. In Proceedings of the Genetic and Evolutionary Computation Conference (GECCO-2008). ACM Press.
Spector, L., J. Klein, K. Harrington**, and R. Coppinger. 2005. Teaching the Evolution of Behavior with SuperDuperWalker. In Proceedings of the 12th International Conference on Artificial Intelligence in Education (AIED-2005), pp. 923-925. IOS Press.
Spector, L., and A. Robinson**. 2002. Multi-type, Self-adaptive Genetic Programming as an Agent Creation Tool. In Proceedings of the Workshop on Evolutionary Computation for Multi-Agent Systems, ECOMAS-2002, International Society for Genetic and Evolutionary Computation.
Crawford-Marks**, R., and L. Spector. 2002. Size Control via Size Fair Genetic Operators in the PushGP Genetic Programming System. In W. B. Langdon, E. Cantu-Paz, K. Mathias, R. Roy, D. Davis, R. Poli, K. Balakrishnan, V. Honavar, G. Rudolph, J. Wegener, L. Bull, M. A. Potter, A. C. Schultz, J. F. Miller, E. Burke, and N. Jonoska (editors), Proceedings of the Genetic and Evolutionary Computation Conference, GECCO-2002, pp. 733-739. San Francisco, CA: Morgan Kaufmann Publishers. (pdf)
Weisler, S., R. Bellin**, L. Spector, and N. Stillings. 2001. An Inquiry-based Approach to E-learning: The CHAT Digital Learning Environment. In Proceedings of SSGRR-2001, the International Conference on Advances in Infrastructure for Electronic Business, Science, and Education on the Internet. Scuola Superiore G. Reiss Romoli, L'Aquila, Italy. Proceedings ISBN: ISBN:88-85280-61-7, URL: http://www.ssgrr.it/en/ssgrr2001/papers.htm.
Spector, L., H. Barnum, H.J. Bernstein, and N. Swamy**. 1999. Finding a Better-than-Classical Quantum AND/OR Algorithm using Genetic Programming. In Proceedings of the 1999 Congress on Evolutionary Computation, pp. 2239-2246. IEEE Press.
Spector, L., and A. Alpern**. 1995. Induction and Recapitulation of Deep Musical Structure. In Working Notes of the IJCAI-95 Workshop on Artificial Intelligence and Music. pp. 41-48.
Spector, L., and A. Alpern**. 1994. Criticism, Culture, and the Automatic Generation of Artworks. In Proceedings of the Twelfth National Conference on Artificial Intelligence, AAAI-94, 3-8. Menlo Park, CA and Cambridge, MA: AAAI Press/The MIT Press. (pdf)
Spector, L., M. J. Rattermann, and K. Prentice**. 1994. Ordering Relations in Human and Machine Planning. In Proceedings of the Twelfth National Conference on Artificial Intelligence, AAAI-94, 80-85. Menlo Park, CA and Cambridge, MA: AAAI Press/The MIT Press.