Artificial General Intelligence: Why Aren’t We There Yet?
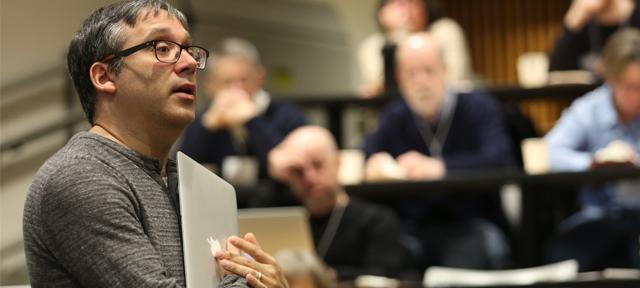
Alum Gary Marcus 86F gave the following lecture as part of Neilfest, an event celebrating the legacy of Psychology Professor Neil Stillings in April. Stillings will conclude his legendary career in June.
I was 16 years old, really not loving high school. I loved what I was doing outside of high school, which was computer programming and thinking about cognitive science. So I decided to drop out and go straight to college. I wrote to a bunch of schools, and Hampshire sent me a little booklet, an excerpt of a cognitive science text. The cognitive science book that Neil put together hadn’t quite come out yet — this was 1986. I came for a visit on January 27, and I know that because on January 26 the Patriots had lost the Super Bowl. To tell you how little I knew of Hampshire, I thought that game might be a problem, that maybe it wasn’t the best timing for an interview. It turned out no one at Hampshire cared about football. (It was later that I discovered the bumper sticker HAMPSHIRE COLLEGE FOOTBALL: UNDEFEATED SINCE 1970.) Over lunch I had conversations with two professors — Mark Feinstein and Steve Weisler — about cognitive science and linguistics. That was pretty exciting for a 16-year-old, and I knew I had found my college.
For decades people have been predicting that artificial intelligence is about 20 years out. They predicted that in 1950 — and in the 1960s. Somebody actually did a historical review of all the predictions, always 20 years away. I think of the Smurfs — “Are we there yet?” they keep asking, and Papa Smurf keeps saying, “Not far now.” And Kurzweil is standing by his prediction that AI is coming. I’m talking about strong AI. Not just AI that can help you find a better ad to sell at a particular moment, but the kind of AI that would be as smart as, say, a Star Trek computer, able to help you with arbitrary problems. Kurzweil still thinks that’s happening in 2029. Andrew Ng, of Stanford, takes a similarly optimistic view. “If a typical person,” he wrote in Harvard Business Review in December, “can do a mental task with less than one second of thought, we can probably automate it using AI either now or in the near future.” Using the cognitive science skills vested in me by this very institution, let’s analyze Ng’s claim. Can we get AI to do what people do in one second? Here are some experiments. Can you describe Image A in a second?
You probably wouldn’t have trouble saying this is a group of young people playing Frisbee, right? Here’s another one, Image B, that’s not too hard.
It’s a person riding a motorcycle on a dirt road. Most of you could do that cognitive task in a second. Here’s one that’s a bit more difficult, Image C.
Most of you could figure it out eventually. It’s a no parking sign with stickers all over it. But here’s what Google’s captioning system came up with, which was shown on the front page of the New York Times. And it’s right out of the Oliver Sacks Hall of Fame: a refrigerator filled with food and drinks. It’s an example of what I call a Long Tail Problem. When you’ve seen a lot of pictures of kids playing Frisbee, you do pretty well in these systems. But when there aren’t a lot of parking signs with stickers in your database, the systems don’t really work. Here’s another one, Image D.
If you’re AlexNet, which everybody in the field has been talking about for the last few years, then you say it’s a school bus. (Everyone’s so excited that neural networks have been solving this thing called ImageNet, but if you take them slightly out of their game, they just fall apart completely.) Here’s another one, Image E.
You think this is a digital clock, right? Of course you don’t. But you would if you were a contemporary AI system called Deep Learning, which is good at categorization but much more limited than most people recognize. You can train it to distinguish Tiger Woods from a golf ball, and probably also from Angelina Jolie. And that’s great. It’s an example of using big data to get statistical approximations. And there’s something called a convolutional network, which was developed by my NYU colleague Yann LeCun, which has enormous practical application in both speech and object recognition. But just because it does a little piece of cognition doesn’t mean it does all of cognition. Here’s another example, Image F, that I think would be quite challenging for the current systems.
First of all, recognizing there’s a dog here would be difficult because you don’t usually see ears in that orientation. And even if you rotated the image, it’s not that typical a dog. I’d guess AlexNet would have some trouble with it. In any case, even if it could identify the dog and the barbell, it’s not going to be able to tell me what you could tell me — which is, it’s really unusual to see a dog doing a bench press. You might wonder, “Has it been taking classes?” You make a lot of inferences about what’s going on, not just identify the parts. So your perceptual system is pretty far beyond where current AI is.
•••
People talk a lot about the exponentials: that transistors have gotten smaller and cheaper, that machines have gotten faster. But if you look at AGI, artificial general intelligence, and if we plot the data for it, the bad news is there is no data for it because nobody’s agreed on the measure for what it would be. So I’ve made up some data to at least illustrate what you might imagine is the pace of the field.
This is Eliza, Image G, next page, which I learned about before coming to Hampshire. It was a computer psycho-analyst that you could basically text message with. People would tell it their problems, and some of them would be fooled by it. But most wouldn’t be fooled for very long. And now we have Siri, which is a little bit better. Talk to Siri about movie ratings and sports scores, and it usually does pretty well. But when you move outside the predefined domains that they’ve had dozens of linguists working on for several years, it doesn’t work that well anymore. Siri hasn’t, I would argue, been real progress in artificial general intelligence.
We actually have some scalable AI now — Google search is scalable AI, using techniques to figure out what you might be looking for, and ad recommendation is scalable AI that you’re subject to when you do a Google search or go to Amazon. But at the same time, the applications are still limited.
They have speech recognition that works particularly in quiet rooms with native speakers, and they’re making some progress in noisy rooms. And image recognition works with a limited set of objects, but not when it’s open-ended. (You can get systems to recognize that those stripes I showed you earlier are associated with a school bus, but they’re not intelligent enough to say no, that’s not really a school bus, just a texture that one would associate with a school bus.) But we still don’t have open-ended conversational interfaces, which we imagined in the 1950s would be here by now. When I was growing up in the 70s, I just assumed we would have solved that one by now.
People have been talking about automated scientific discoveries for years, but I don’t think there’s been real progress on that except in narrow slices of the problem, like identifying if one gene is similar to another gene. Automated medical diagnosis is still struggling. There’s been a lot of progress in radiology, for example, where it’s based on an image, but AI is still pretty weak once you have to combine ideas, text, different symptoms, and so forth. You could imagine, in fact Facebook has imagined, automated scene comprehension for blind people. But if you try out any of these apps, they’re limited. They can recognize a wineglass, but can’t recognize a wineglass in a dark restaurant with forks around it. We all know Rosey the Robot. Okay, everyone my age who knows The Jetsons knows Rosey, a kind of arbitrary domestic robot. (Now that I have two kids, I would pay a lot of money for such a thing. Babysitters in New York — kind of dicey to find them on short notice — but Rosey would always be available. That would be awesome . . . )
We still don’t have domestic robots we could remotely trust. We don’t have driverless cars we can trust.
Lately, AlphaGo is probably the most impressive demonstration of AI. It’s the AI program that plays the board game Go, and extremely well, but it works because the rules never change, you can gather an infinite amount of data, and you just play it over and over again. It’s not open-ended. You don’t have to worry about the world changing. But when you move things into the real world, say driving a vehicle where there’s always a new situation, these techniques just don’t work as well. If all we want AI to do is target advertisements, then problem solved. But if we don’t advance AI, then we may never build machines that can read open-ended text. Or cure cancer. Because cancer involves so many different proteins, probably no human being can understand all that’s going on. We’ll want to couple the power of computation with the resourcefulness of human scientists, and we don’t know how to do that yet. We might never be able to understand the brain for the same reason, if we can’t build better AI.
I opened this talk with a prediction from Andrew Ng: “If a typical person can do a mental task with less than one second of thought, we can probably automate it using AI either now or in the near future.” So, here’s my version of it, which I think is more honest and definitely less pithy: If a typical person can do a mental task with less than one second of thought and we can gather an enormous amount of directly relevant data, we have a fighting chance, so long as the test data aren’t too terribly different from the training data and the domain doesn’t change too much over time. Unfortunately, for real-world problems, that’s rarely the case.
My biggest fear about AI right now is that it’s actually getting stuck. This is what we call a local minimum. The idea is that you’re trying to get to the bottom of the hill, and you keep going down, taking small steps. If you have a smooth surface, that works. But if you have a complicated surface, you might get stuck in one valley when you really want to get below it. I have the sense that the AI field might be doing just that. On some tasks, I think we actually are getting to the bottom, like object recognition and speech recognition. We keep taking little steps toward doing better and better on these benchmarks, like recognizing a set of a thousand objects. We also might be getting close to theoretical best performance in speech recognition and object recognition, maybe language translation. But in other areas, I’m not sure that this technique is getting us to the right place. Like language understanding: it’s not clear to me that we’re making any real progress at all.
The risk is we could spend a lot of time on the task of AI and eventually only hit a wall. That depends again on what we’re trying to do. If AI recommendations for advertisements are 99.975 percent correct, for example — that is, they’re wrong one time out of 40 — it’s not a big deal. (To put it another way: If AI says, well, because you liked this Gary Marcus book you’ll like this other one, and you hate it, it’s just too bad.) But if AI does that for a pedestrian detector and one time out of 40 is wrong, that’s a whole other issue. Same with domestic robots interacting with live humans. If you have an elder-care robot that puts your father in the bed 39 times but on the 40th time it misses, this is obviously not . . . optimal.
Why aren’t we there yet? I think one of the problems is that engineering with machine learning, which has become the dominant paradigm, is really hard. It’s difficult to debug it. The paradigm is that you have a lot of training examples. You see inputs; you have outputs. But you don’t know if when you get to the next set of data it’s going to be like the data you’ve seen before. There are wonderful talks by Peter Norvig in which he describes this in some detail. There’s also a paper by D. Sculley and others, “Machine Learning: The High-Interest Credit Card of Technical Debt.” The idea is, it works on your problem and when your problem changes even a bit, you don’t know if it’s going to continue to work. You incur “technical debt.” You could easily be out of luck later.
The second issue, I think, is that statistics is not the same thing as knowledge. All apparent AI progress has been driven by accumulating large amounts of statistical data. We have these big correlational models, but we don’t necessarily understand what’s underlying them. They don’t, for example, develop common sense.
Image H is the cover from an article I wrote recently with Ernie Davis on common sense: the robot sawing away on the wrong side of the tree limb. You don’t want to learn that that’s not the proper technique by having 10,000 trials or a million trials — this is not a big data problem. You want to have a very small amount of data and a very small amount of error.
Another issue is nature versus nurture. In the AI field, people seem to want to build things that are based completely on nurture and not on nature. In my talks I often show a video of a baby ibex climbing down a mountain. It can’t be that the baby ibex has had a million trials of climbing down. You can’t say that trial-by-trial learning is the right mechanism for explaining how well the ibex is doing there. You have to say that over evolutionary time, we’ve shaped some biology to allow the creature to do this. In the spirit of comparison, this would be the state of the art for robots. To accomplish this I would look more to interdisciplinary collaboration (the way I learned at Hampshire) and to human children. For example, I have two kids, Chloe, who is two years and ten months old, and Alexander, who is four. They do a lot of things that are popular machine learning, but they do them a lot better through active learning and novelty seeking. They have fantastic common-sense reasoning and natural language. I think we should be trying to understand how kids do that. I’ll give you just one more example.
A few weeks ago Chloe gave me a piece of artwork she made, and I said, “Let’s put it in Mama’s armoire.” Here’s what Chloe said, two years ten months old: “That way Mama doesn’t see it, but when she opens it she can see it.” Let’s take a look at that utterance. It’s fairly syntactically sophisticated for her age. She’s inferring the intent behind Papa’s (that’s me) plan. She’s figuring out why I would want to do this, and then she’s able to articulate a complex idea in verbal language. She understands a potential future that she hasn’t directly observed. She doesn’t need the data, doesn’t need to see Mama encounter the artwork in the armoire. She gets the general idea.
For comparison, let’s ask Siri, “What happens when you put artwork in an armoire?” And the answer is, “Okay, I found this on the Web for what happens when you put artwork in an armoire.” The first hit from Bing is what happens if you break an artwork, which is really not what I was talking to Chloe about.
I’ll end with a rhetorical question: Isn’t it time we learn more from human beings? This is, of course, exactly the kind of question I learned to ask here at Hampshire, with Neil Stillings as my Div II and Div III adviser.
Gary Marcus 86F, scientist and entrepreneur, was a cofounder and the CEO of Geometric Intelligence, a machine-learning start-up recently acquired by Uber. Trained by Neil Stillings and MIT’s Steven Pinker, he’s an award-winning professor of psychology and neural science at New York University. He’s also the best-selling author of a number of books (Guitar Zero, Kluge, The Future of the Brain). He makes frequent appearances on radio and television.
From the Summer 2017 issue of Hampshire's Non Satis Scire magazine.